INSIGHTS

8 min read
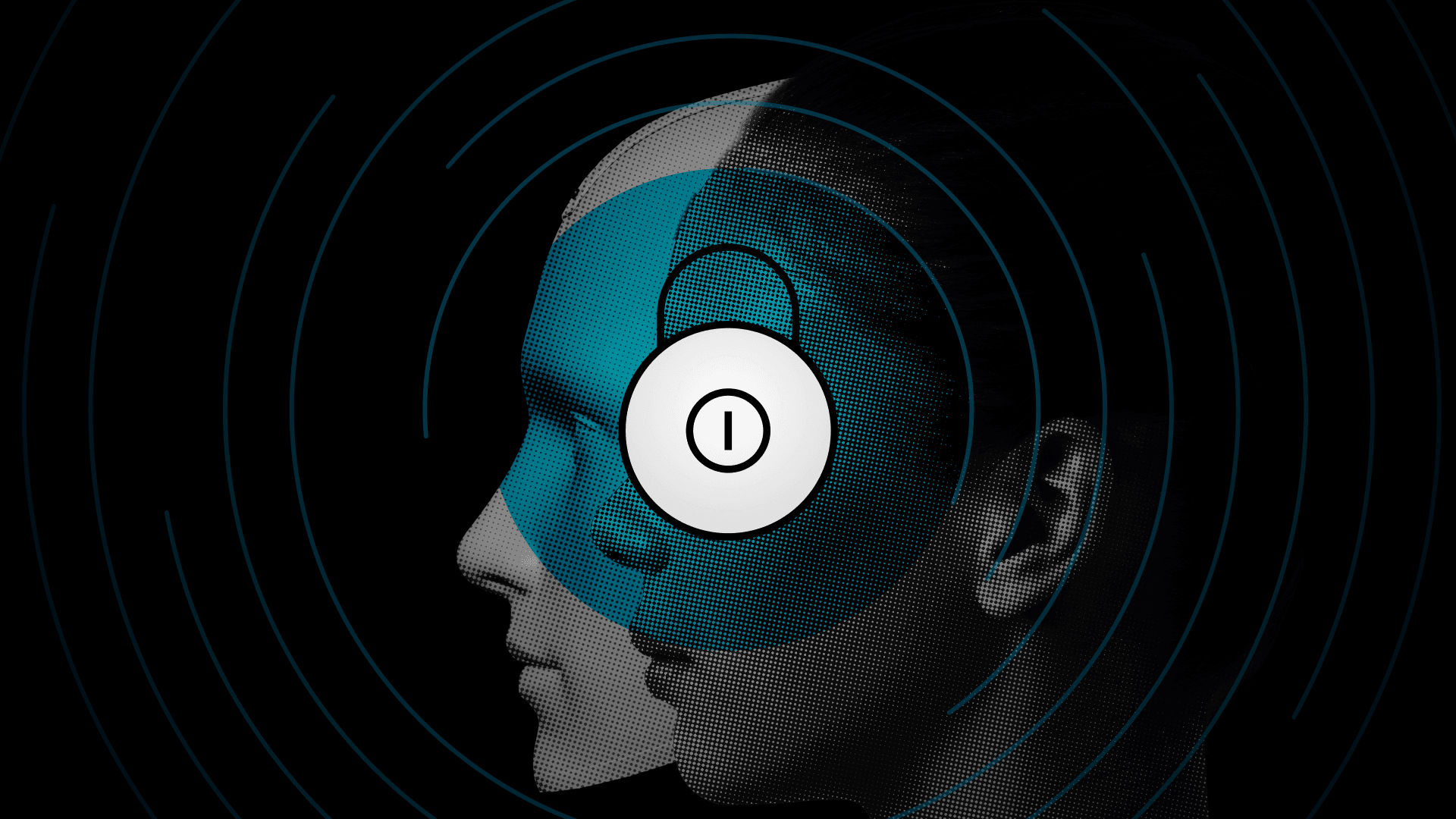

Share
Published on 10/03/2023
Last updated on 02/09/2024
AI or security? Yes, your enterprise needs both.
Share
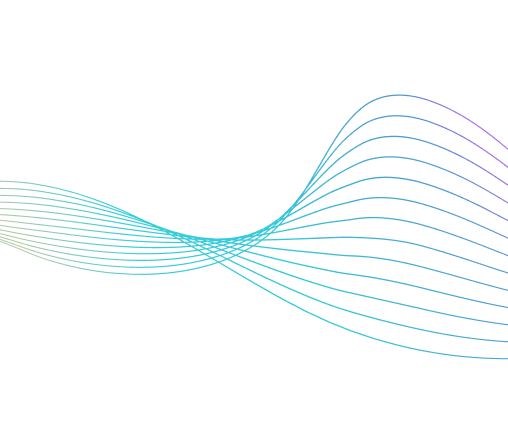
Get emerging insights on emerging technology straight to your inbox.
In our previous article, we looked at how the integration of AI in your enterprise puts the issue of customer trust at the very center of your concerns. Perhaps you’re crafting optimal, personalized patient care plans. Or maybe you’re generating influencer content based on customer data trends.
At the enterprise level, there are so many ways to capitalize on the tremendous potential of AI. But how are you using your customer data? Are you transparent with them about that usage? Is the data stored securely? Are your AI models protected from tampering?
If your enterprise pursues AI integration, then you must be acutely aware of the corresponding security implications. The task before you is much greater than the creation of efficient models and algorithms. If customers are to trust your AI-powered solutions, then you owe it to them to prioritize privacy, authenticity, and attribution.
For enterprise stakeholders, trustworthy AI is not just a technical asset; it is a promise of secure and responsible AI operations. The bottom line is this: Your enterprise cannot have AI without prioritizing security. To do so would introduce a level of risk that most enterprises and their stakeholders cannot stomach.
In this post, we’ll look closely at security challenges of AI integration, considering how enterprises can secure their systems and data in response.
We’ll start by addressing one of the first stages in the AI journey: data collection.
Data collection and dealing with sensitive data
Effective AI starts with data collection. Perhaps you’re gathering log data from components across your distributed system, or you’re gathering biometric data from participants in a pharmaceutical drug trial. Regardless, the more data you can gather and use to train your AI models, the higher the quality of your models’ predictions and outputs.
The Problem: During the data collection phase, you run the risk of unintentionally gathering sensitive information—personal identifiable information from customers, confidential business data, proprietary research information, and more.
If you don’t handle this sensitive data appropriately, it will become a vulnerability. Unauthorized access to this data could lead to:
- Breaches of data privacy
- Legal and financial repercussions from compliance violations
- Loss of customer trust and damaged brand reputation
The Solution: Your enterprise can adopt the following practices to help protect against the improper gathering, storage, or incorporation of sensitive data:
- Implement strict data governance policies to oversee what data is collected and how it is used.
- Perform regular data audits to identify any unintentional collection of sensitive data.
- Employ data classification techniques to ensure that sensitive data is immediately recognized and handled with the necessary care.
Model training and the exposure of confidential data or IP
As we move further along the pipeline of AI processes, we arrive at model training. We’ve considered the unintentional inclusion of sensitive data in the data collection phase, but what about the case of sensitive data that is meant to be incorporated? Often, datasets used for model training include confidential or proprietary information out of necessity.
The Problem: When training datasets are shared or accessed, intentionally included confidential data or intellectual property (IP) might be exposed.
When IP or confidential data is exposed, competitors might learn of your business strategies or gain an undue advantage. Confidential data that is incorporated into your AI models without proper handling may expose your enterprise to industrial espionage.
The Solution: Various data privacy techniques can be applied to help protect confidential training data from inadvertent exposure. These techniques include:
- Leverage tools to apply differential privacy, ensuring the model does not memorize specific details from training data while preserving the accuracy of model outcomes.
- Segregate sensitive or confidential data with data partitioning to ensure that it’s not used in shared or public models.
- Implement anonymization practices to de-identify data, thereby minimizing the impact if a data breach does occur.
Ensuring secure models
Even after your data has been properly collected, handled, and secured, your AI models themselves are susceptible to security threats.
The Problem: Malicious attackers can try to compromise the integrity of your model and its results through the following attacks:
- Model poisoning: Inserting misleading or incorrect data into a training dataset in order to compromise a model’s predictive accuracy or behavior
- Model tampering: Altering an already trained model by accessing and modifying a model’s parameters, architecture, or configuration settings
- Introduction of bias: Skewing a model’s decision-making by manipulating training data, features, labels, or feedback loops
We can’t overstate the repercussions of an AI model with skewed or malicious outputs, especially if the model is used to guide critical business decisions or to interact with end users.
The Solution: Ultimately, securing your AI models depends on proper validation and monitoring:
- Enforce trusted data sourcing through data lineage tracing and quality checks to guarantee that training data is genuine.
- Conduct regular model validation to ensure that a model is performing as expected and to detect abnormalities in its outputs.
- Implement continuous model monitoring—including its infrastructure and network—to provide real-time insights into its health and functioning, enabling rapid detection - and mitigation of any anomalies.
Securing your stored data
As we’ve noted, integrating AI into your enterprise requires massive amounts of data, necessitating massive data storage solutions.
The Problem: Stored data (both raw and processed) can be an attractive target for malicious actors, especially given its volume and potential value.
If your data storage solutions are brittle or insecure, your enterprise is susceptible to data breaches that could expose sensitive user or business data. A data breach could lead to substantial financial losses, legal consequences, and damaged business reputation.
The Solution: Your enterprise can put into place several measures to secure its stored data:
- Data encryption is your foremost defense, ensuring that stored data—even if it is accessed without authorization—remains unreadable.
- Use cloud storage solutions that enact security measures that prevent common data breach threats.
- Perform regular backups of your data.
- Implement robust access control measures to protect access to critical datasets.
Safeguarding your tools and processes
Certainly, integrating AI in your enterprise will require the adoption of various third-party tools.
The Problem: Third-party dependencies, such as open-source tools, might have security vulnerabilities that attackers will exploit in order to compromise your AI processes.
If your tools are compromised, then the result could be corrupted AI models or skewed results. Exploited dependencies may even become a backdoor for further breaches into your enterprise’s infrastructure.
The Solution: Enterprises can take proper actions to ensure the security of their software supply chain.
- Ensure that all tools come from trusted sources. Regularly update those tools to ensure they have the latest security patches.
- Use software composition analysis (SCA) to gain insight into the potentially vulnerable, open-source components used in your pipeline.
- Adopt the use of software bill of materials (SBOMs) coupled with vulnerability scanning to achieve robust software supply chain security.
Building trust = prioritizing security
The transformative potential of AI for enterprises is undeniably powerful. However, the integration of AI in the enterprise demands an approach that prioritizes trustworthy and responsible AI. This means establishing a strong commitment to security.
We’ve looked at how integrating AI into your enterprise introduces points of vulnerability. Whether it’s the incorporation of sensitive data into training sets or malicious attackers trying to tamper with your models or tools, your enterprise must take a proactive and informed approach to secure its data and systems.
As the world continues to move toward AI-centric solutions with increasing fervor, resources like Outshift are shaping global discussions and practices in order to promote trustworthy and responsible AI. And Outshift stands ready to support you as you move forward in this journey, ensuring that your AI adoption is as secure as it is innovative.

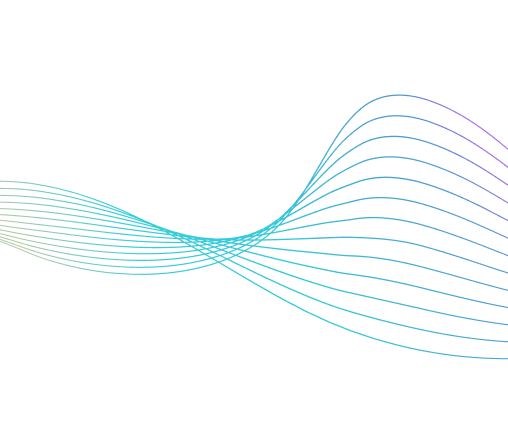
Get emerging insights on emerging technology straight to your inbox.
Unlocking Multi-Cloud Security: Panoptica's Graph-Based Approach
Discover why security teams rely on Panoptica's graph-based technology to navigate and prioritize risks across multi-cloud landscapes, enhancing accuracy and resilience in safeguarding diverse ecosystems.
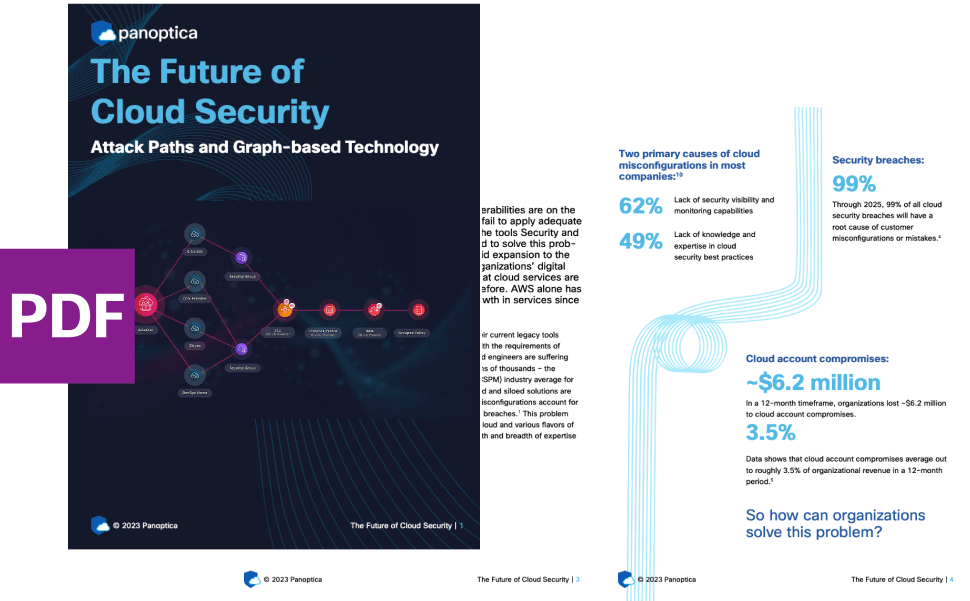
Related articles

The Shift keeps you at the forefront of cloud native modern applications, application security, generative AI, quantum computing, and other groundbreaking innovations that are shaping the future of technology.
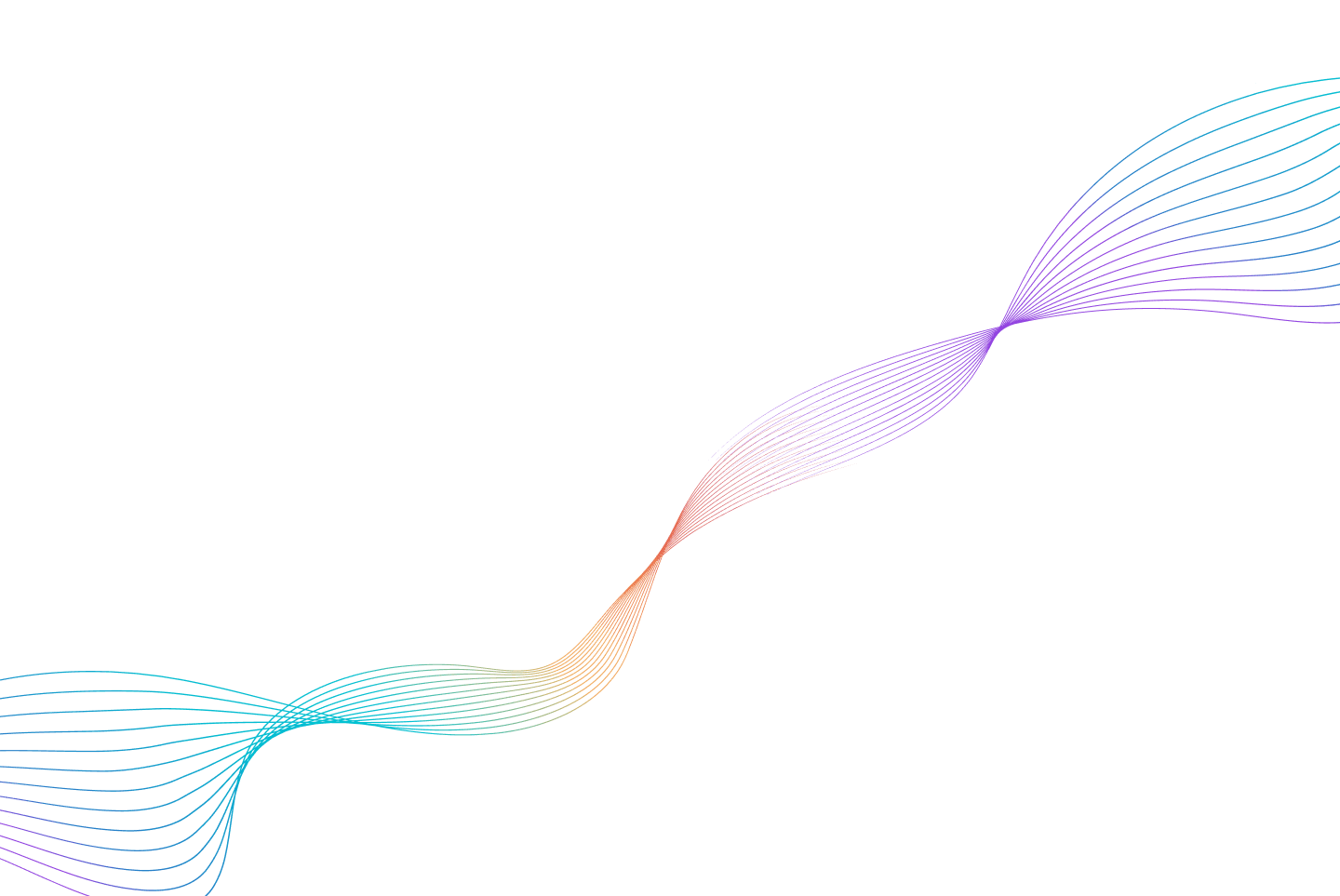