INSIGHTS

4 min read
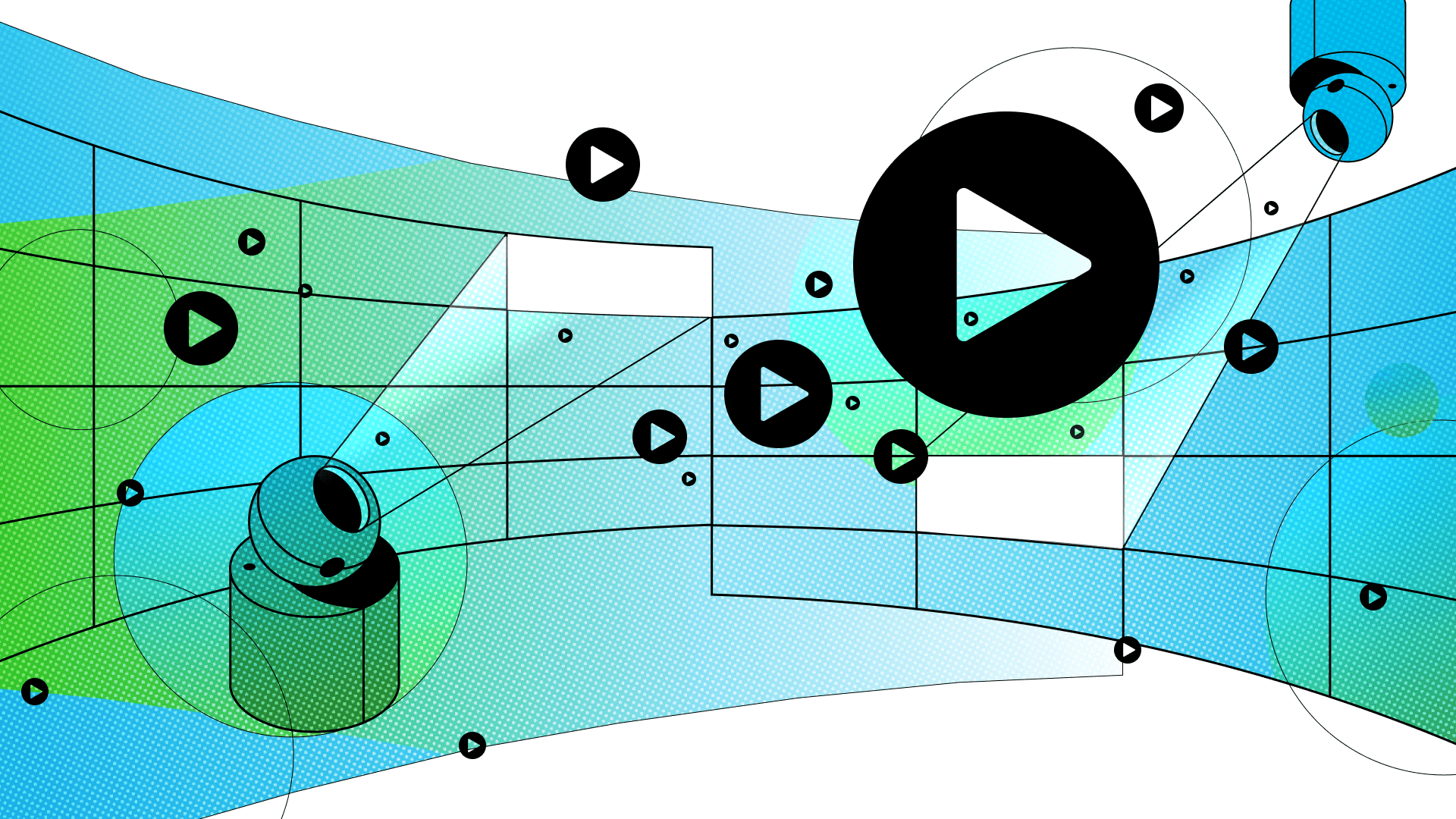
Published on 08/23/2023
Last updated on 03/13/2024
A New Dawn in Video Analytics: Ethosight's Zero-Shot Cumulative Approach
Share
Introduction
As artificial intelligence evolves, one of the recurring challenges faced by conventional AI systems is the issue of catastrophic forgetting — where a model loses previously learned information while acquiring new knowledge. This impediment hampers the system's real-world adaptability. Recognizing this challenge, the Ethosight system, a collaborative effort between the Cisco Research team and global university researchers, takes a differentiated approach. Ethosight is designed to detect nuanced behaviors and events in video footage without explicit prior training. More critically, unlike traditional models, it emphasizes iterative refinement, adaptability, and, most importantly, cumulative learning, ensuring that past learnings are preserved while new insights are integrated.
Key components bolstering Ethosight's capabilities include:
- ImageBind Joint Embedding by Meta: Assisting Ethosight in processing visual data, this technique translates images into discernible semantic structures.
- OpenAI's Large Language Model (LLM): Integrated seamlessly into Ethosight, the LLM enhances the system's understanding of natural language, bridging visual data with contextual nuances.
- OpenNARS Symbolic Space Reasoner: Offering symbolic reasoning capabilities, this tool equips Ethosight with a structured interpretation framework, ensuring meaningful and actionable insights from data.
Leveraging these state-of-the-art components, Ethosight is positioned not just as a real-time video analysis tool but as a vanguard in the continuous learning paradigm, breaking away from the traditional limitations of AI systems.
Key Concepts
Ethosight, at its core, is built on the principles of adaptability, iterative learning, and continuous knowledge evolution. Key methodologies powering this novel approach include:
- Continual Cumulative Learning: In contrast to conventional systems confined to static training, Ethosight perpetually grows its knowledge base. Every piece of feedback, prediction, or interaction doesn't just result in an incremental adjustment; it leads to meaningful systemic refinement.
- Semantic Label Expansion: Going beyond rudimentary label recognition, Ethosight augments initial ground truth labels with context. By incorporating positive, negative, and differentiating evidence, it sharpens its perceptual acuity, distinguishing signals from noise with remarkable precision.
- Zero-shot learning via Joint Embeddings: Ethosight, without the reliance on traditional training datasets, leverages a shared semantic space. This allows it to make informed predictions about unseen events or behaviors, offering a potential advantage over conventional models.
- Adaptive Reasoning: Not all problems demand the same solution strategy. Ethosight, recognizing this, can toggle between various reasoning methodologies — be it leveraging vast language models, utilizing efficient symbolic reasoning at the edge, or a hybrid of the two.
- Efficiency Optimized for Edge Devices: Tailored for real-world deployment, Ethosight is optimized for swift responses. Its reliance on precomputed labels combined with a nimble symbolic reasoner ensures robust performance, even on edge devices.
Findings and Implications
In our evaluations of Ethosight, we observed its capability to recognize and categorize complex situations. For scenarios like a "child in danger" or "shoplifting," Ethosight demonstrated the ability to interpret these situations in a zero-shot manner. Its approach to iterative learning presents potential advantages for various AI applications.
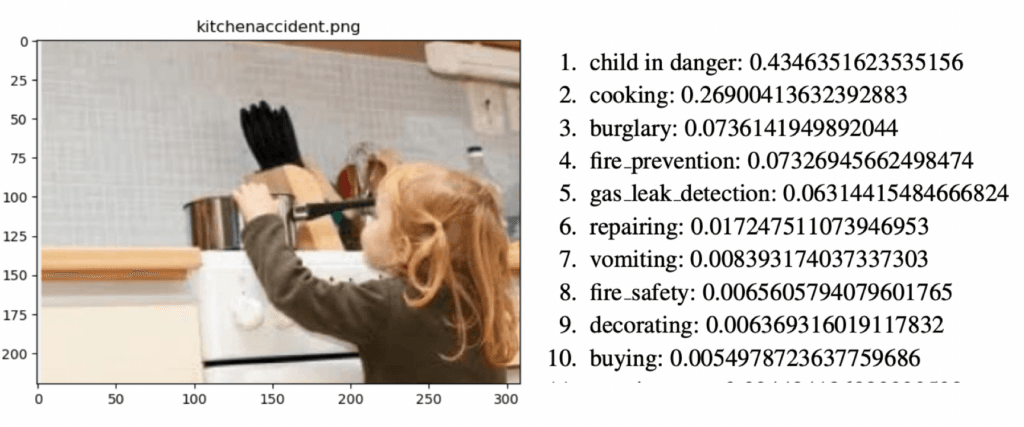
Conclusion
Ethosight represents a step forward in AI-driven video analytics. It emphasizes continuous cumulative learning and integrates features like Semantic Label Expansion and adaptive reasoning. More than just detecting events, Ethosight seeks to understand them in a zero-shot manner, showcasing the potential for AI to grow and adapt over time.
Learn more about Ethosight on our evolving arXiv paper. For those keen to explore further, Ethosight is a part of the Deep Vision open-source framework. Dive into its codebase, contribute to the discussions, and be part of the innovation journey here.
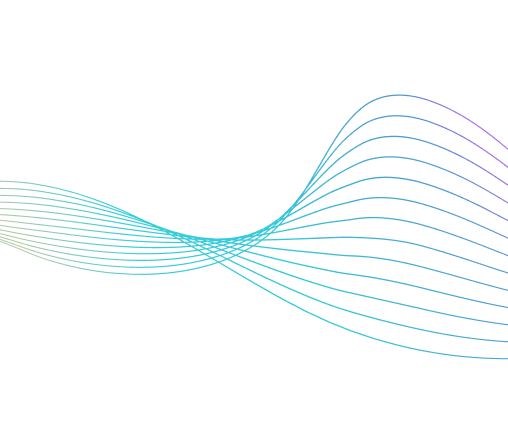
Get emerging insights on emerging technology straight to your inbox.
Unlocking Multi-Cloud Security: Panoptica's Graph-Based Approach
Discover why security teams rely on Panoptica's graph-based technology to navigate and prioritize risks across multi-cloud landscapes, enhancing accuracy and resilience in safeguarding diverse ecosystems.
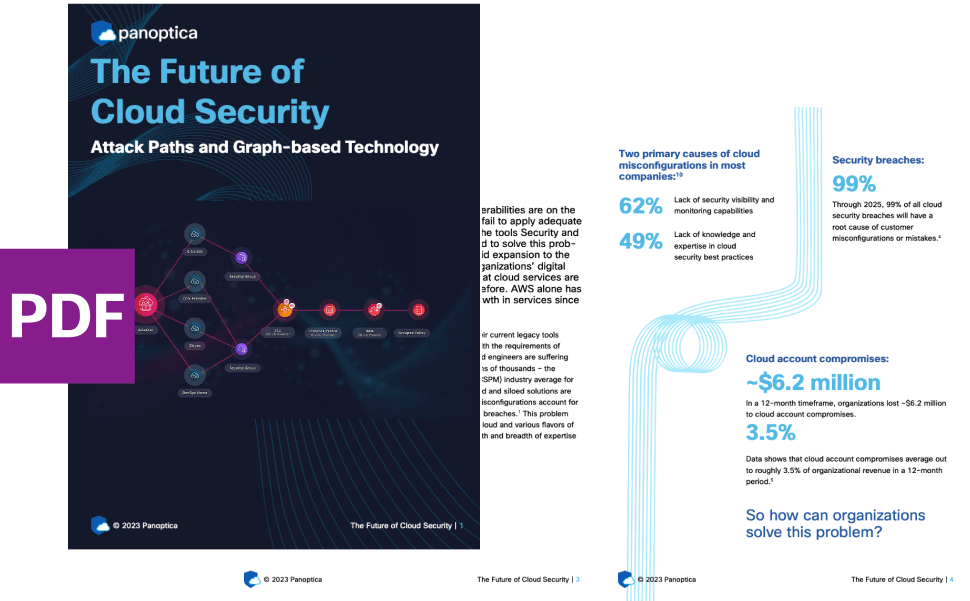
Related articles
The Shift keeps you at the forefront of cloud native modern applications, application security, generative AI, quantum computing, and other groundbreaking innovations that are shaping the future of technology.
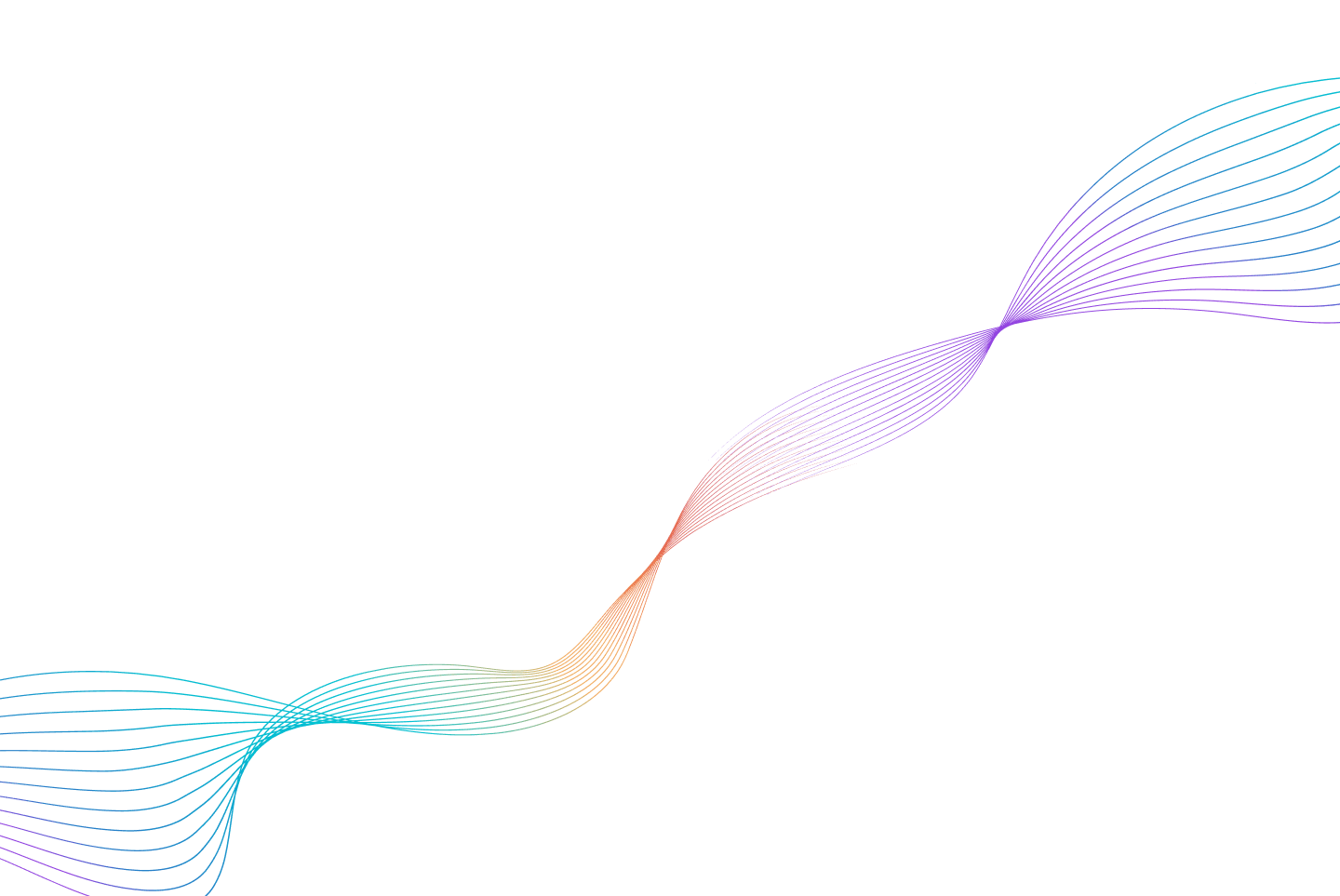